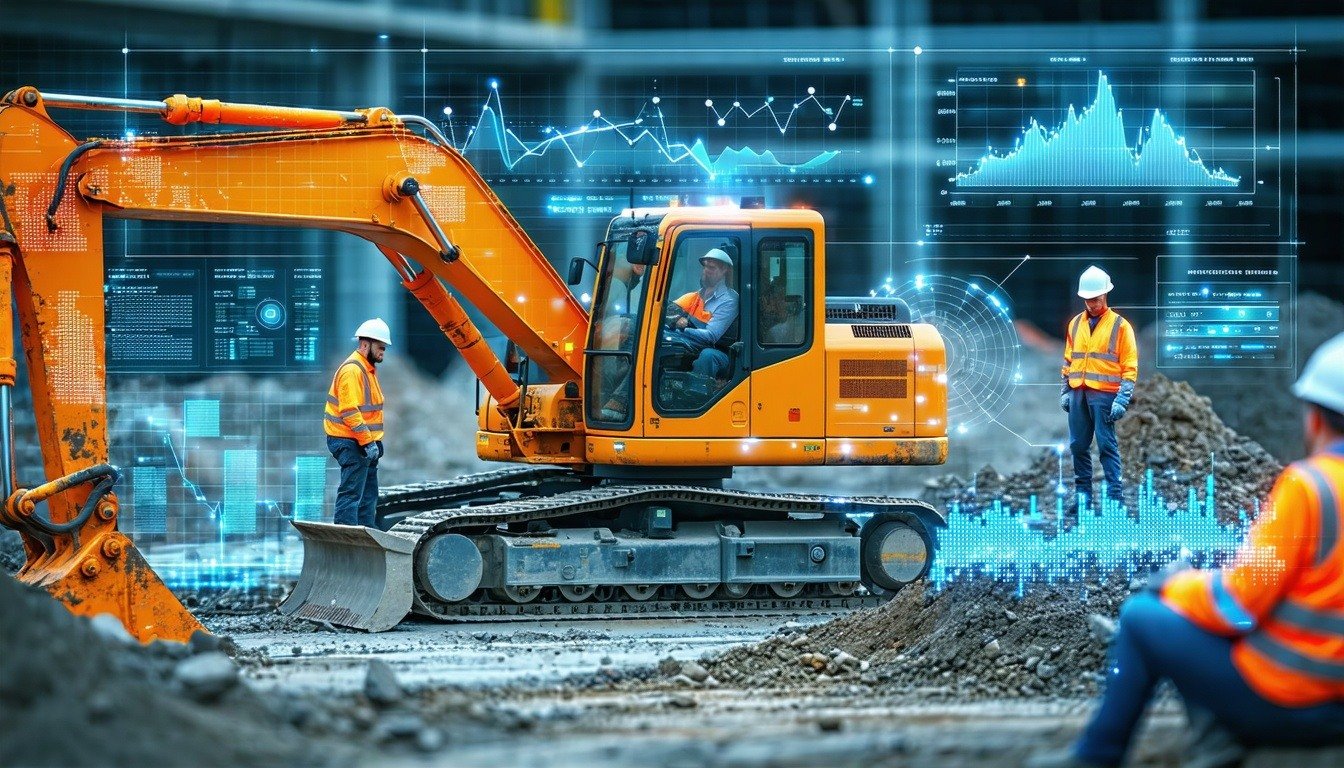
Unlock the potential of predictive analytics in construction with Azure Machine Learning to forecast job profitability and make data-driven decisions.
The Importance of Predictive Analytics in Construction
Predictive analytics is revolutionizing the construction industry by providing builders and contractors with powerful tools to anticipate project outcomes and enhance decision-making processes. By leveraging historical data and advanced algorithms, predictive models can forecast job profitability, enabling companies to allocate resources more effectively and mitigate risks.
In an industry where margins are often thin, the ability to predict financial performance is invaluable. Predictive analytics helps identify potential cost overruns, delays, and other issues before they become critical, allowing for proactive management and improved project outcomes. This technological advancement is driving a new era of efficiency and profitability in construction.
Getting Started with Azure Machine Learning: A Builder's Guide
Azure Machine Learning (Azure ML) provides a comprehensive platform for building, training, and deploying predictive models. For builders and contractors new to this technology, getting started can seem daunting. However, Azure ML offers a user-friendly interface and a wealth of resources to simplify the process.
First, create an Azure account and navigate to the Azure Machine Learning Studio. Here, you can set up a workspace, which will serve as the central hub for your machine learning projects. Azure ML supports a variety of data sources, including cloud storage and on-premises databases, making it easy to integrate your existing data into the platform.
Collecting and Preparing Data for Predictive Modeling
Data is the foundation of any predictive model. For construction projects, relevant data may include historical job costs, timelines, resource allocation, accounts payable information from tools like Buildertrend, and other financial metrics. It's crucial to gather comprehensive and accurate data to ensure the reliability of your predictions.
Once collected, the data must be cleaned and preprocessed. This involves removing any inconsistencies, filling in missing values, and transforming the data into a format suitable for analysis. Azure ML provides various tools and techniques for data preparation, streamlining this critical step in the modeling process.
Training Your Predictive Model: Step-by-Step Process
With your data prepared, the next step is to train your predictive model. Begin by selecting an appropriate algorithm based on the nature of your data and the specific prediction goals. Azure ML supports a wide range of machine learning algorithms, including linear regression, decision trees, and neural networks.
After selecting an algorithm, split your data into training and testing sets. The training set is used to teach the model, while the testing set evaluates its performance. Azure ML provides tools to automate this process, ensuring that your model is both accurate and reliable. Once trained, the model can be fine-tuned using various optimization techniques to improve its predictive power.
Leveraging Predictive Insights for Enhanced Project Management
The true value of predictive analytics lies in its application. By integrating predictive insights into your project management processes, you can make more informed decisions and optimize project outcomes. For example, predictive models can help identify which projects are likely to experience cost overruns or delays, allowing you to allocate resources more effectively and take corrective action early.
Additionally, predictive analytics can enhance financial planning and reporting. With accurate profitability forecasts, you can set more realistic budgets, improve cash flow management, and ultimately increase the financial stability of your construction business. The integration of tools like Buildertrend with Azure ML can further streamline these processes, providing a cohesive and efficient workflow.